Research studies often fall prey to experimental bias, in which the results are not representative of what they are supposed to measure. This limits the applicability of the results to anything beyond the experiment itself, which decreases or eliminates the value of those results.
External Validity
A study that is externally valid is one in which the data and conclusions gathered from the results of an experiment can be applied to the general population outside of the experiment itself. If the study's data and conclusions cannot be applied to the general population, including general events or scenarios, then the experiment's results are only relevant to that experiment, and nothing more. A study's external validity can be threatened by such factors as small sample sizes, high variability, and sampling bias.
Small Sample Sizes
The smaller the sample size for an experiment, the less applicable the results will be to the general population. The world has some 7 billion individuals, and thus a representative sample in any experiment would have to be very large to be applied to this general population. Nonetheless, the larger the sample group is in relation to the general population to whom the results are to be applied, the more likely it is to be applicable.
This premise, however, can be negatively impacted by the law of diminishing returns, which states that effectiveness will decline after a certain amount of success has been achieved. This means that after a certain point, including more individuals in a study would gradually have less value to researchers. This could be caused by a multitude of factors, including cost and time put into the research. Generally it is best to attain a reasonable sample size that is representative of the population being studied.
High Variability
Variability, also known as dispersion or spread, refers to how spread out a group of data is, or how much the measures differ from each other. Data sets with similar values are considered to have little variability because the values are within a smaller spread, whereas data sets with values that are spread out have high variability because the values are within a larger spread. In many instances of high variability there are outliers, which are values that exist far outside of the area where the majority of values are found. In many cases these outliers, which increase the variability of the data set, are removed when conducting statistical analysis of the data.
Sampling Bias
Sampling bias occurs when the sample participating in the study is not representative of the general population. This may be the result of purposeful selection of participants by the researcher, but there are many other factors that can create sampling bias. One example is surveys taken during a presidential election. The results of the surveys often depend on the city, state, or area being surveyed. For example, people in cities tend to vote one way, while people in rural environments often vote another. Similarly, one's geographic location (the Northeast, South, Midwest, etc.) can have an impact on who is being surveyed. If there is a high saturation of a given political party in an area surveyed, then the results will be skewed in the direction of the political party, and not be representative of the general population.
Selection Bias
Selection bias happens when the comparisons in data from the sample population have no meaning or value because the participants in the sample were not equally and fairly selected for both the experimental and control groups. Both the experimental and control groups should be representative of the general population, as well as representative of each other. One group should not show substantially higher characteristics of a given variable than the other, as this can distort the findings.
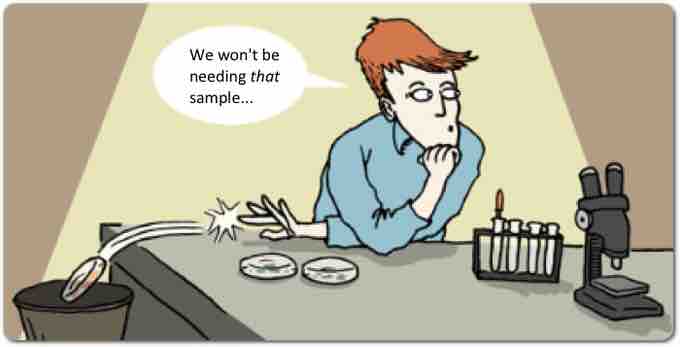
Research bias
When conducting research, sample and selection biases can impact the results of the research. This will impact whether the data is externally valid, meaning that it can be applied to the general public.
Response Bias
Response bias (also known as "self-selection bias") occurs when only certain types of people respond to a survey or study. When this occurs, the resulting data is biased towards those with the motivation to answer and submit the survey or participate in the study. The resulting data, however, is not representative of the desired sample, nor the population at large. This is because only a select few have answered the survey and participated in the experiment. This data requires a disclaimer saying that out of all respondents, a certain characteristic is found. Regardless of a disclaimer, the results cannot be applied to the general population, nor the entire desired sample group.
For example, imagine that a university newspaper ran an ad asking for students to volunteer for a study in which intimate details of their sex lives would be discussed. Clearly, the sample of students who would volunteer for such a study would not be representative of all of the students at the university (many of whom would never want to volunteer for such a study due to privacy concerns). Similarly, an online survey about computer use is likely to attract people more interested in technology than is typical. In both of these examples, people who "self-select" themselves for the study are likely to differ in important ways from the population the experimenter wishes to draw conclusions about. Thus, the responses collected are biased and not representative of the general population of interest. Many of the admittedly "non-scientific" polls taken on television or websites suffer from response bias.
A response bias can also result when the non-random component occurs after the potential subject has enlisted in the experiment. Considering again the hypothetical experiment in which subjects are to be asked intimate details of their sex lives, and assume this time that the subjects did not know what the experiment was going to be about until they showed up. When they found out, many of the subjects would refuse to participate, leaving only those students who are very interested in discussing their sex lives, which results in a biased sample.
Reliability
Another important issue to consider when collecting data is reliability. Reliability refers to the overall consistency of a measure. This means that any surveys, tasks, or measures the researcher administers during a study need to produce similar results each time they are used under similar conditions. If a measure is not reliable, it will produce different results, even under the same conditions. Consider a scale that measures how much you weigh. If one day the scale shows that you weigh 150 lbs yet the next day it shows you 170 lbs, it may be time to shop for a more reliable scale. Let's look at another example. A researcher is running a study using a questionnaire that assesses emotion— specifically negative affect. If the emotion questionnaire produces completely different results, even when very similar participants with identical levels of negative affect under identical experimental conditions complete the questionnaire, it is not reliable, and the data cannot be trusted. Ideally, the two similar participants with identical levels of negative affect should score very similarly on the emotion questionnaire. This would indicate the measure's ability to produce consistent results under similar conditions: the measure would be considered reliable.
Therefore, when determining the reliability of a measure, a researcher must determine how much variability is stemming from measurement error (assumed to be random error) and how much is stemming from the "true score" or the actual, replicable aspects of the phenomenon being measured. This concept is sometimes referred to as "classical test theory." Researchers typically do this by pre-testing their measures on preliminary samples of participants, and by running descriptive reliability analyses that indicate to them the measure's overall consistency.