Forecasting in Accounting
In corporate finance, investment banking, and the accounting profession, financial modeling is largely synonymous with cash flow forecasting.
This usually involves the preparation of detailed company specific models used for decision making purposes and financial analysis.
A financial forecast is an estimate of future financial outcomes for a company or country (for futures and currency markets). Using historical internal accounting and sales data, in addition to external market and economic indicators, a financial forecast is an economist's best guess of what will happen to a company in financial terms over a given time period—usually one year.
Challenges
Arguably, the most difficult aspect of preparing a financial forecast is predicting revenue. Future costs can be estimated by using historical accounting data; variable costs are also a function of sales.
Forecasting vs. Financial Plans and Budgets
Unlike a financial plan or a budget, a financial forecast doesn't have to be used as a planning document. Outside analysts can use a financial forecast to estimate a company's success in the coming year.
Forecasting is the process of making statements about events whose actual outcomes (typically) have not yet been observed. A commonplace example might be the estimation of some variable of interest at some specified future date. Prediction is a similar, but more general term. Both might refer to formal statistical methods employing time series, cross-sectional or longitudinal data, or less formal judgmental methods.
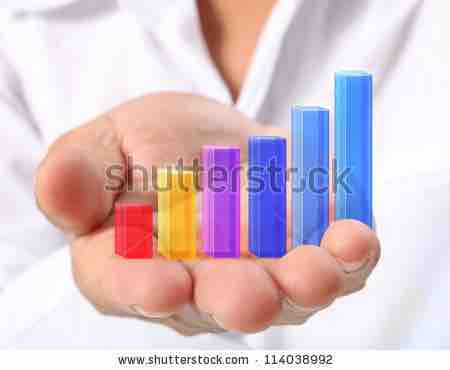
Forecasts are company specific and can either show negative or positive trends.
Predicting revenue can be challenging. Investors use forecasting to identify positive revenue predictions, like in this picture, for good investments.
Time Series Data
Time series is a sequence of data points, measured typically at successive time instants and spaced at uniform time intervals. Examples of time series are the daily closing value of the Dow Jones index or the annual flow volume of the Nile River at Aswan. Time series analysis comprises methods for analyzing time series data in order to extract meaningful statistics and other characteristics of the data. Time series forecasting is the use of a model to predict future values based on previously observed values. Time series are very frequently plotted via line charts.
Cross-sectional data
Cross-sectional data refers to data collected by observing many subjects (such as individuals, firms or countries/regions) at the same point in time, or without regard to differences in time. Analysis of cross-sectional data usually consists of comparing the differences among the subjects.
For example, if we want to measure current obesity levels in a population, we could randomly draw a sample of 1,000 people from the population (also known as a cross section of that population), measure their weight and height, and calculate what percentage of that sample is categorized as obese. For example, 30% of our sample may be categorized as obese based on our measures. This cross-sectional sample provides us with a snapshot of that population, at that one point in time. Note that we do not know based on one cross-sectional sample if obesity is increasing or decreasing; we can only describe the current proportion. Cross-sectional data differs from time series data also known as longitudinal data, which follows one subject's changes over the course of time. Another variant, panel data (or time-series cross-sectional (TSCS) data), combines both and looks at multiple subjects and how they change over the course of time. Panel analysis uses panel data to examine changes in variables over time and differences in variables between subjects.
Longitudinal Data
A longitudinal study is a correlational research study that involves repeated observations of the same variables over long periods of time — often many decades. It is a type of observational study. Longitudinal studies are often used in psychology to study developmental trends across the life span, and in sociology to study life events throughout lifetimes or generations. The reason for this is that unlike cross-sectional studies, in which different individuals with same characteristics are compared, longitudinal studies track the same people, and therefore the differences observed in those people are less likely to be the result of cultural differences across generations. Because of this benefit, longitudinal studies make observing changes more accurate, and they are applied in various other fields. In medicine, the design is used to uncover predictors of certain diseases. In advertising, the design is used to identify the changes that adverts have produced in the attitudes and behaviors of those within the target audience who have seen the advertising campaign.
Judgmental methods
Judgmental forecasting methods incorporate intuitive judgements, opinions and subjective probability estimates, such as Composite forecasts, Delphi method, Forecast by analogy, Scenario building, Statistical surveys and Technology forecasting.
Usage of forecasting can differ between areas of application: for example, in hydrology, the terms "forecast" and "forecasting" are sometimes reserved for estimates of values at certain specific future times, while the term "prediction" is used for more general estimates, such as the number of times floods will occur over a long period.